Tom Hanika
Visiting Scientist (level W2) at University of Hildesheim
Research Associate (on leave) at University of Kassel
Temporary Lecturer at Humboldt-Universität zu Berlin
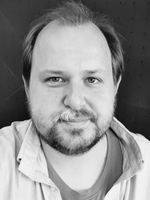
My research is concerned with the foundations and applications of explainable artificial intelligence. I am particularly interested in the (interactive) extraction of knowledge from explicit and implicit data by means of machine learning procedures. At the very heart of my work is the study of semantics arising from implicational theories in complex and large data. Of special interest in this context are lattice orders and ordinal-metric spaces. Problems in high dimensions are of special interest to me, as they represent a current challenge for the effectiveness of learning procedures. Apart from that I am very interested in unsupervised learning from large text corpora as well as applications of my methods in various research fields, such as Biology, Geography, Physics, Digital Humanities, and more.
Besides my work in research I am also a senior developer of the social publication sharing system BibSonomy as well as maintainer of the FCA-tool conexp-clj.